A Holistic Approach to the Ethics of AI for Fraud Prevention
- Luciana B
- Dec 13, 2024
- 5 min read
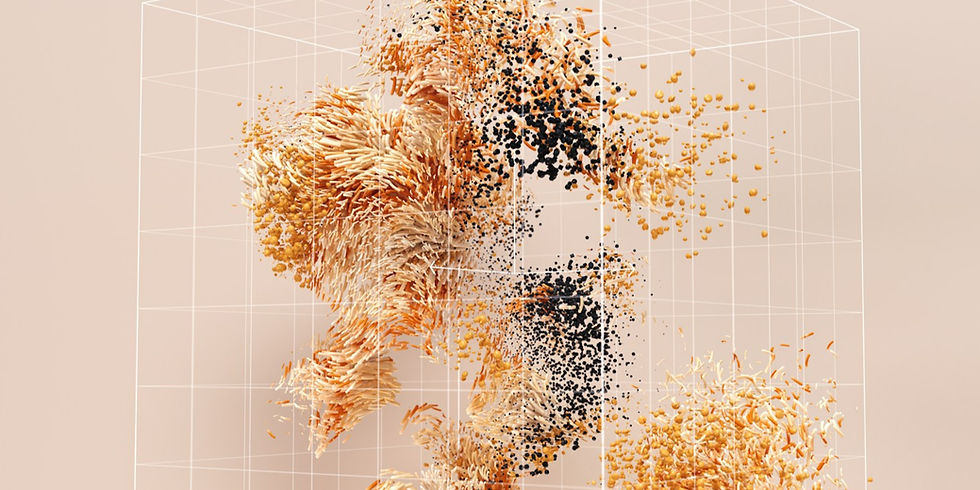
Author: Amber Devin
In a time where artificial intelligence (AI) is reshaping and revolutionising the way the financial sector attempts to tackle fraudulent activity, the discussion of ethical fraud prevention is often left aside. This article will outline the various ethical concerns with the use of AI for fraud preventative purposes, before highlighting and suggesting the need for a more holistic approach to be applied when dealing with the ethical concerns of AI and machine learning within financial industry.
Section 1: How is AI used for fraud prevention?
You may be wondering, how exactly AI is used within financial sectors for fraud prevention. In simple terms, AI systems have the ability to sort through inconceivably large datasets, with high levels of accuracy in identifying irregular patterns or abnormal behaviours that indicate fraudulent activity. The system is then able to flag the transaction, and block it, before the fraud happens, or can continue. Over time, and the more you use your bank card, the AI is able to detect normal patterns of purchasing behaviour specific to the user, meaning fraud is less likely to successfully occur.
Section 2: Ethical concerns
So, what’s the problem? Using AI for fraud prevention seems like a really good thing... which it is! But there are still significant ethical concerns that need to be discussed and considered when using this technology.
The main ethical concerns with the use of AI for fraud prevention in the financial sector are, algorithmic bias concerns; transparency and black box problems; accountability concerns; data privacy and security concerns; and accuracy concerns. Currently these concerns are, in both academic literature and policy viewed as very separate concerns that, whilst all are important, they must be dealt with individually. Later in this article we will dispute this, but firstly we will outline each of the key concerns.
Algorithmic Bias Concerns refer to when programs manifest the biases found within their data. Often this occurs due to certain groups of people (usually minority groups including women, or people of colour) being underrepresented within training datasets, or due to pre-existing prejudices coded into the data itself resulting in unfair and biased decisions and outcomes. Since AI systems are often trained on historical data, often historical prejudices can seep through and become amplified by AI systems. For instance, due to women not being legally able to apply for loans until 1975, there is not enough historical training data showing women being approved for loans. An AI system may take this, and assume therefore, that women should not be approved for loans at the same rate as their male counterparts, making it harder for women to access loans since the AI system responsible for making decisions on loan approvals is biased towards women due to a bias pre-existing in its training data, thus resulting in biased decisions by the algorithm on the basis of gender.
Transparency in AI systems is deemed essential to maintaining customer trust and credibility within financial industries. AI system’s decisions about what is and isn’t fraud need to be transparent in order to be rationalised and understood to prevent unfairness and discriminatory practice. However, the black box problem causes serious concern for transparent AI systems. The black box problem refers to the phenomena that sometimes an AI’s decision or outcome cannot be understood or rationalised – how the AI arrived at that conclusion is unknown – despite it not being absurd. We will come back to this in section 3.
Accountability concerns arise when an AI system makes a wrong, or unjust decision. Who should be held accountable for the mistake of the AI system? If anyone? For instance, it is massively inconvenient for your card to be frozen due to suspected fraud in the case of a false positive – and sometimes may lead to an innocent person being investigated for fraud. Who should be held accountable for this if the decision was made by an AI system?
This leads to another concern: accuracy. AI systems need to be highly accurate. They must catch fraud whilst simultaneously not flagging every transaction as fraudulent. They need to be able to, in seconds, detect abnormal and fraudulent activity, whilst not alerting false positives. This is hugely important for customer trust within financial sectors.
Finally, the last ethical concern we will explain is data privacy and security concerns. With AI systems, needing to be trained on large, and diverse training datasets, involving the use of countless amounts of personal data, ethical concerns over the user’s data privacy (in terms of ownership, misuses, consent, fairness) and security (who has access, safety) arise.
Now that all the main ethical concerns when considering the use of AI systems for fraud prevention in financial sectors have been defined individually, we will now explain why a holistic approach to their solution is necessary for their solution, despite not being considered or advocated for hugely within literature or policy documentation.
Section 3: Benefits of a holistic approach
These concerns, whilst typically thought of as independent ethical concerns are hugely interlinked. A holistic approach, we argue, must be applied in order to reach their solution.
Firstly, what do we mean by a holistic approach? By a holistic approach we mean, rather than considering each of these concerns as individual problems, they need to be considered by a comprehensive method since they are all relevantly interlinked as we will show after explaining this approach. A holistic approach recognises the interconnectedness of all these key concerns, focusing on a long-term sustainable perspective and preventative focus. For instance, in fields such as healthcare a holistic approach would treat the whole person, not just the symptoms. In terms of ethical AI for fraud prevention in financial sector we can imagine that each of these concerns are simply the symptoms of the ‘whole’, and it is the ‘whole’ we must treat.
So, how are these concerns interlinked?
Each of these concerns are interlinked through either way of confliction or way of mitigation of the other.
For instance, through high levels of transparency, accountability and algorithmic bias concerns can be mitigated, since the route of the bias can be accounted for and rectified and the responsible party (e.g. the programmer, training data, etc) can be identified. However, on the flip side of this, high levels of transparency conflict with high levels of accuracy, two things identified of particular importance. High degrees of accuracy in AI systems require complex AI models that cannot be fully understood by humans, meaning highly accurate AI models cannot be hugely transparent. Since accuracy is so important for preventing false positives and identifying fraudulent activity, but transparency is so important for preventing algorithmic bias and thus, discriminatory, or unfair practice, a confliction interlinking the two concerns can be made. This, we argue, emphasises the need for a holistic approach to solving these problems. There are several more conflictions including; a confliction between accuracy and data privacy, due to the amount of large datasets filled with personal data needed to train accurate AI models; and conflictions between the mitigation of algorithmic biases and data privacy and security concerns, since the use of large, diverse training data sets is necessary to avoid the risk of algorithmic bias problems, whilst simultaneously raising concerns over data security and privacy.
Thus, we argue a holistic approach, emphasising balance, considering the bigger picture, recognising these interconnections and links - whilst drawing from a multi-disciplinary perspective - is necessary to ensure the ethical use of AI for fraud preventive purposes.
Komentáře